Wednesday 19 july 2023
Artificial intelligence (AI) is a formidable tool for data analysis, one capable of processing vast amounts of data generated from the natural world. As such, many practitioners in the field of nature conservation are finding ways to use AI to solve some of the problems present in this field. This article explores how AI could be used by Civil Society Organisations (CSOs) to improve the governance of tropical forests and build resilience to various threats.
Header photo: Nambian desert © Mickael Tournier for Unsplash
Text: Peter Adema
We classify the available AI solutions and show some real-world use cases of the usage of AI for locally-led forest governance. Access to innovative technology such as AI is highlighted as an important step forward within the Kunming-Montreal Global Biodiversity Framework, to meet the needs for effective implementation of the goals and targets of the framework, particularly in low- and middle-income countries (target 20). We therefore consider it timely and relevant for our CSO partners to enable and support them in adopting these technologies into their day-to-day work.
What are the five classes of AI solutions for nature conservation?
1. Assessment: Streamlining data analysis
Identifying and assessing the biodiversity within a given area has historically posed significant challenges for nature conservation and ecology. CSOs working on nature conservation must know the types and population counts of species within conserved regions to determine the presence of endangered species or launch new conservation programs. Advancements in technology now allow for the remote collection of ecological data using various sensors, such as camera traps and microphones placed in forests and images from satellites or drones. However, the subsequent analysis of the recorded information, including species identification, presents a significant hurdle.
Fortunately, AI can simplify this process by automatically transforming images and recordings into usable information. By offloading much of the analysis work to AI, organisations can focus on taking the necessary actions to preserve nature.
Real-world applications where AI has allowed organisations to safely analyse data on a larger scale include taking an inventory of forests in Armenia, finding animals in hundreds of thousands of images in Palawan and counting species in the rainforest of the Congo basin.
2. Monitoring: Enhancing surveillance capabilities
In addition to assessing the current state of nature reserves, CSOs must also vigilantly monitor these areas for potential disturbances, such as poaching or illegal logging. However, limited human resources often prevent comprehensive monitoring across vast nature reserves. Modern technology enables the deployment of remote sensors to cover expansive land areas.
Nonetheless, constant human surveillance of these sensors can prove arduous or unfeasible for CSOs that must work on a tight budget. Here, AI solutions can come into play, automatically filtering out irrelevant data and directing human attention to potential issues within the nature reserve.
Examples of how this can be implemented include large-scale audio monitoring in Peru by Rainforest Connection, satellite image analysis to find illegal logging in Paraguay by Guyra Paraguay and video monitoring of national parks to ensure visitor safety in Korea.
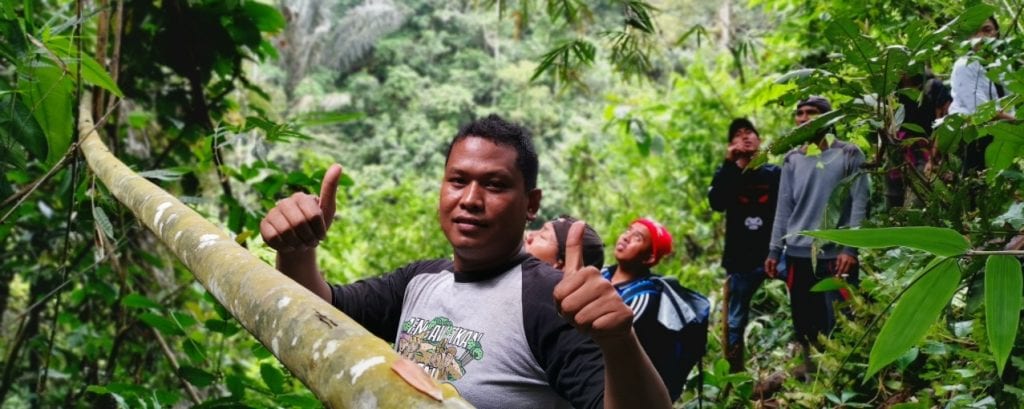
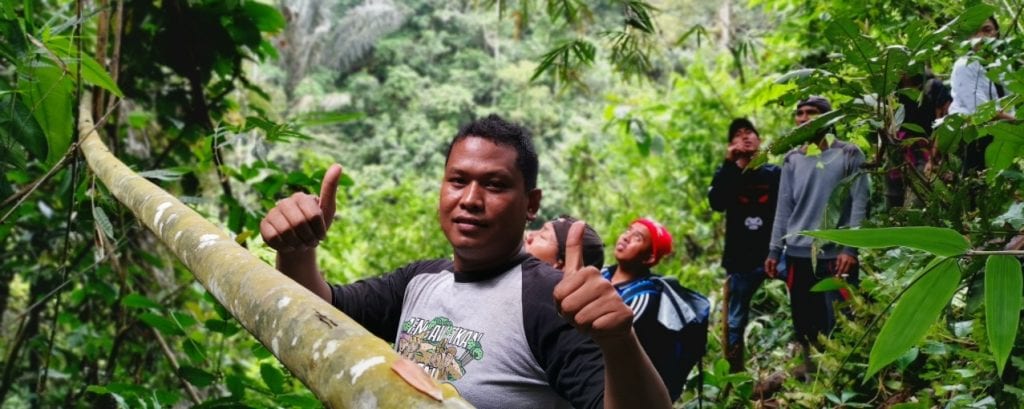
3. Prediction: Proactively addressing threats
Efficient resource allocation within larger areas necessitates CSOs’ understanding of potential threats within nature reserves, such as poaching, deforestation, or natural issues. Predicting these problems poses challenges for experienced nature conservationists and scientists due to the countless factors involved. AI can significantly assist CSOs in this area.
Although many predictive models have specific scopes, focusing on deforestation risks or simulating the various impacts of climate change scenarios on species survival, they often outperform human capabilities within those domains. This is thanks to their ability to effectively calculate risks based on vast amounts of information.
Examples of successful implementation of predictive AI include WWF’s Forest Foresight accurately predicting logging operations in Gabon and Kalimantan, wildfire prediction in Australian forests, and projection of likely logging sites in the Amazon, Brazil.
4. Decision-making: Facilitating informed choices
While many CSOs have a clear mandate to protect specific lands, there are regions where the exact boundaries of nature reserves and parks need clarification. Determining these boundaries often involves balancing species preservation, the needs of inhabitants, and available budgets. CSOs working on locally led forest governance face significant challenges due to numerous factors and extensive regions involved in such decisions.
One emerging application of AI lies in assisting with this complex decision-making process. By creating models of the area and considering all available data, programs can suggest viable courses of action that align with given budgets. This can allow CSOs to determine courses of action that allow for the preservation of as much nature as possible, given constraints such as budget and the interests of other stakeholders.
Examples of using these planning tools include finding trade-offs between land owners, palm oil producers and natural preservation in Kalimantan, developing policies for protecting the Coral Triangle and determining suitable reservation sites for the forests of Guyana.
5. Communication: Overcoming language barriers
Many conservation organisations operate in countries where English is not the primary language, leading to communication barriers when engaging with international counterparts. While translators, both human and digital, can mitigate this issue to some extent, generative AI offers a compelling alternative in numerous cases. Generative AI encompasses models that generate text or other content based on prompts. Text models (such as ChatGPT) can aid non-English organisations in international communications by providing corrections, rephrasing sentences, or summarising complex texts.
Moreover, models like Midjourney can create images, assisting in designing supporting visual content. It is important to note that generative AI models, unlike analytical AI variants, have no accuracy guarantees. CSOs considering using models like ChatGPT should note two characteristics of current models. Firstly, that it is necessary to provide all relevant information to ensure accuracy, as the model’s self-generated information may be unreliable. Secondly, the models are currently very inexpensive or even free to use due to the companies behind them wanting to scale up. As such, we would suggest CSOs to explore how they could use generative models before prices rise.
What are the potential downsides of AI?
While the previous paragraphs highlighted various areas where AI can aid nature conservation, it is crucial to acknowledge the potential downsides associated with its usage. Primarily, the resource costs of running AI models locally can exceed the budgets of smaller organisations, necessitating centralised hardware infrastructure.
This centralisation could be provided by larger programmes such as the Green Livelihoods Alliance, or by the companies responsible for the AI models. Selecting partners for centralisation should be done with care, however, as organisations providing centralisation are often able to view all usage of the AI model, raising privacy concerns for many organisations. Moreover, running these models consumes significant amounts of electricity, impacting the climate footprint of the CSO.
Furthermore, operationalising custom models requires the assistance of external experts, which can be difficult to find in low- and middle-income countries and comes with a high start-up cost. Finally, the generative AI models discussed earlier may not be freely available in the future, leading to further expenses.
A valuable tool
Artificial intelligence holds great promise for improving the conservation and sustainable use of ecosystems values in a rapidly changing and resource-limited world. AI tools are becoming increasingly valuable for CSOs for effective governance of natural areas, including tropical forests.
These tools enable data analysis, remote surveillance, prediction of threats, prioritisation of areas for conservation and improve communication. Challenges exists in building capacity and ensuring access to AI tools, but we believe that the long-term benefits will outweigh these challenges and AI will become a valuable tool in many conservation efforts.